Do we really want machines to recognise us? Credit: Scott Barbour/Getty Images
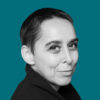
After achieving her childhood dream of going to MIT, Joy Buolamwini tried to build an art project called “The Aspire Mirror”. The device she designed would greet her face every morning by projecting a different, inspiring image onto her reflection. A fearless lion’s face, for example. The trouble was, the off-the-shelf facial recognition program Buolamwini was using didn’t even recognise her face as a face. To get the Aspire Mirror to work, she had to don a white plastic mask.
That was the beginning of her voyage of discovery into the biases of computer code; one which would — as a new Netflix documentary, Coded Bias, shows — lead her to testify before lawmakers in the U.S. Congress. Facial recognition technology (FRT), Buolamwini proved, is often poor at identifying black faces and female faces — largely because it is “trained” on data sets with far more images of white men. The bias may be inadvertent, but, as Buolamwini points out, has very real consequences.
Still, in a biased world, do you really want FRT to perform well in recognising your face? If you’re trying to unlock your phone, probably yes. If you want to project a fearless lion’s face onto your reflection in the morning, I suppose so. But if you’re walking past a Metropolitan Police FRT van, possibly not. In Coded Bias, Buolamwini’s story is interwoven with that of Big Brother Watch, as the organisation challenges police use of FRT. In a sequence filmed on London’s streets, a 14-year-old black schoolboy is stopped and searched by plain clothes officers after his face is wrongly matched by FRT with a suspect on their database.
Previous surveys in the UK have found that ethnic minority respondents were less keen for the police to have unfettered use of FRT. Not just because the technology is less likely to wrongly tag a white schoolboy, though that is true. Ethnic minorities’ experience of bias — not their knowledge of the technology — makes them less likely to see the enhancement of police powers as a good thing. More accurate technology, trained on more diverse databases, won’t help that lack of trust in the police.
“What is the purpose of identification?” asks Apartheid historian Patric Tariq Mellet when Buolamwini visits South Africa. Mellet displays the racial classification papers that allocated South Africans to categories — based not on who they felt themselves to be, but what the state decided they were. The purpose of this identification was clear: to control where the carrier could go, what they could do, even whom they could marry.
With facial recognition technology, there is no need to carry papers. Your face is your ID card. You could be sorted by any system, not just by visible characteristics like skin colour or sex, but by linking your face to all the unseen information sitting in databases. Not only your credit history, but your internet search history. Not just your home postcode, but your mood as expressed in walking speed.
Join the discussion
Join like minded readers that support our journalism by becoming a paid subscriber
To join the discussion in the comments, become a paid subscriber.
Join like minded readers that support our journalism, read unlimited articles and enjoy other subscriber-only benefits.
SubscribeThe way in which the majority of the population blithely accepts the destruction of our liberties and the imposition of mass surveillance etc is profoundly depressing. I just wish the rest of us could somehow set up a new country somewhere.
There are 3 things we can do
But yes, it is profoundly depressing to realise that we’re surrounded by morons.
I think I’m already adopting 2. To misquote… “switch off, tune out, drop out”. if you just accept that 99% of the population are gullible idiots who believe anything the BBC / CNN tells them, and learn to just ignore the prattle and bleating, life becomes much more pleasant.
The good news is that CNN is in freefall and most people no longer believe the BBC. For instance, Don Lemon on CNN only got 600K viewers the other night, half that of the excellent Greg Gutfeld’s new show on Fox, which it was up against.
CNN”s viewing figures have more or less halved since Trump left, and they were pretty bad prior to that. Meanwhile, Tucker Carlson on Fox keeps getting bigger and bigger and people like Tim Pool, Ben Shapiro and Steven Crowder continue to attract millions of views on YouTube etc.
The average age of a CNN viewer in the US is about 67. In general, nobody below the age of about 50 has any interest in anything the MSM has to say. They know that the MSM is garbage and/or lies.
My remainiac, (formerly) BBC loving Dad actually cancelled his TV licence last week in protest at the fear mongering output. When it’s content is so irrelevant even to its core demographic, then you know they’re really in trouble!
I might have been characterised as ‘remainiac’ back in 2019, but as the EU has failed to defend liberal democracy over the last year or so, I no longer care about it. I’ve long found the BBC problematic, but would have got a licence had the Olympics not been postponed. Now, even if they go ahead I don’t think I could bring myself to contribute to what is now a relentless propaganda machine.
That’s encouraging, thank you.
I did pretty much that for years. After a brief period of spoiling the ballot papers I just didn’t bother at all. Brexit brought me back to life and hope, but I’m now minded to switch off again and try to enjoy the last of the summer wine – if only I’m allowed.
I’m OK with 1 and 2; finding 3 very challenging!
So basically hide? Hide in plane sight perhaps, by just not doing the easy things like do not have a cell phone, or get a burner often at least, A VPN (I recommend NORD as they are Panamanian and so keep no records) and never use a camera with a GPS or it marks all your pictures with the location and date stamp, that then will mix with every other picture in the world and you are found. Get a digital camera that does not have one.
But then ‘Why Bother’? We are dull and not breaking the law, so who cares if everything about us is watched and documented?
But the answer is, aesthetics. One pulls the curtains when at home after dark as we are naturally bothered by being watched wile not knowing. We close the door of the bathroom when home alone, even, we do not like being spied on instinctively because it is creepy knowing some creep is hiding behind the wall watching us, just aesthetics, but that is reason enough.
Just hope there are no criminals in your new country. The most obvious example of the surveillance state/society is CCTV cameras. But how many more crimes would go unsolved without CCTV? Given that ‘look at all the CCTV in the area’ is the default police response for many crimes, I’d guess the figure would be high. Against that, what evidence is there that CCTV has been mis-used? I’ve missed it if there is any.
Technology has outpaced our ability to use it wisely and it appears we have either forgotten or ignored one of life’s elemental truths: no matter how wonderful a new tool or invention is, someone will figure out a way to use it for bad purposes.
Perhaps a good start would be to stop treating technology as though it’s a person. The algorithms for FRT are designed by humans; the algorithms that serve as a media big brother are created by humans. “The computer” did not anything other than what some person told it to do.
We have “leaders” salivating to implement a Chinese-style system of social credit through the vaccine “passport.” Who in their right mind thinks the document would begin and end with this jab, a jab that we’re already told requires regular follow-ups? This scheme is designed, and purposely so, to be the proverbial camel’s nose under the tent. It would mark the foundation of a national (global?) database that will only grow, and then use that information to determine who can or cannot participate in random activities that we take for granted in a free society.
But this brings us to the hardest, most important question: why don’t we object more often?
I’m not sure who “we” is but objections about, especially about the passports that are gross invasion of personal privacy and more than a little creepy. We already know that govt spies on us regularly; we already know that big tech collects information on us for the purpose of selling us things; we already know that people can scarcely breathe if separated from smart phones for more than eight seconds. The objections are dismissed as the work of tinfoil hat wearing conspiracists.
Every proposal cited from the book is based on coercion, ideas so grand that they must be mandatory. You can believe in the threat of climate change all you want, but businesses being “obliged” and people being “compelled” sounds like a dystopian novel.
Here is the question that the warmists seldom address: why should any rational person believe that the same people who have made a mess of public education, who mismanaged the pandemic, who cannot handle a budget, and who apparently hire racist cops are the people we should entrust here? It’s hard to imagine a greater display of hubris than the belief among elected officials and bureaucrats that they are uniquely qualified to manage the climate through policy.
“Every proposal cited from the book is based on coercion, ideas so grand that they must be mandatory.”
Hardly surprising. Every time I see a photograph of Prof Schwab I wonder where his white Persian cat is.
It is sitting on Bill Gates lap purring. And look closely, see the white hairs on the leg of Bezo’s trousers? We are doomed, the Global Elites have decided the Middle Class are to be destroyed as they are the only power base which hold them from utter Dr Evil rule. They are winning. Work From Home is soon to be work from Manila and New Deli and the Middle Class decimated like Stalin did to the Kulaks.
If you like to be “obliged,” “compelled,” “frowned upon,” that’s your privilege. Enjoy. But it seems a cynical and gutless approach, as evidenced also in your later post.
What’s the alternative?
Been banging the drum along these lines for a while. You have a structural problem the nature of which is not fully understood. Existing political governance structures where humans make the decisions, will *never* be able to react to technology driven change fast enough to keep control over technology.
What is likely to happen in response, is that decision making will be increasingly handed over to ever more sophisticated adaptive algorithms, some of this in the name of ‘fairness’, as human decisions will come to be seen as ever more error prone in the face of rising systems complexity.
But this is of course just another form of loss of control.
That’s quite a bold assertion, given that every technology ever invented has been brought under our control. No reason is offered why this may be true. This is simply tech exceptionalism, and is a case usually advanced by large technology companies: “Can’t catch us, we’re so fast!”
I can indeed provide bucketloads of arguments to support my assertion, but it can get tedious if I splurge out an essayfull of stuff. If instead I put forward something terse, it would (legitimately) be challenged.
I will post something as a starter for ten this evening and we can debate further if you wish.
write your essays, I read them, but there is not keeping up and there is encouraging. Like China is pushing ahead in this, it is not bad that free societies push back. Obviously once IA is fully released it will not matter though. AI is going to what AI is going to do.
“every technology ever invented has been brought under our control“ – really? Do you not think our societies are now controlled by cars, computers, the internet, smartphones? These technologies dominate and shape our lives, and there is very little we can do about it. I can choose not to drive, or own a smartphone, but then I can’t physically move, increasingly I can’t do simple things like operate a bank account, book a ticket or buy many essential goods and services
Ok, so there are many ways to show that tech advance is outstripping the ability of traditional human political structures to successfully apply governance, and I will start from the angle of illustrating a couple of specific examples of the ungovernability of tech below.
(i) Bitcoin. Bitcoin came into being over a decade ago when its creator (or creators) conjured up literally tens of billions out of thin air. Yet, we cannot even *identify* the originators or how much of the original holding they are still sitting on, and in which jurisdiction, much less tax them. And how would you prevent ever more sophisticated, and private, counter-currencies from forming that will potentially bypass all auditing and all taxation and threaten the very notion of modern nationhood?
(ii) Pornography. In over two decades of the internet, it has proven impossible to keep online porn out of the reach of minors, even in autocratically governed places like Arabia or (until recently) China. This is not because nations are fond of the idea of allowing access to porn to minors, it is because both prevention and policing are damn near impossible. The UK recently came close to putting into place regulation of the wild west that is the porn industry by requiring age verification, and then backed off. I think it eventually dawned on someone in government that the chances of actually being able to police porn on a global scale to prevent the UK popuation from accessing whatever it likes is… precisely zero.
I can in fact, present dozens of such scenarios, ever more complex, shining a searchlight on the historical nature of money and trade, and the unspoken human scale assumptions that have underpinned all human governance prior to the rise of ubiquitous computation, which have now been blown out of the water by algorithmic technologies – through the unbounded speed and scaling of replication, decision-making, transmission that computation enables. Algorithmic technologies are not human scale and lawmakers without intimate knowledge of tech haven’t got a hope of drafting law quick enough in reaction – and by the time they react the landscape has altered and the tech has moved on, so they are mechanically set to remain behind the curve.
The Chinese have in fact managed to keep a lid on their internal cyberspace remarkably successfully, not by creating ‘rules’, but by operating a high-surveillance dystopia. The distinction is critical. Coercion is always possible, for a period anyway. China’s success is based on the fact that the outside cyberworld has been shut out (The Great Firewall) and that anyone logging on to their internal cyberspace is required to identify themselves via facial recognition tech or other means – everyone and everything tracked in effect. Additionally, the CCP has direct hooks into the companies that produce both the software and the hardware that the majority of Chinese are coaxed into using. It is highly likely that the versions of Android that go on devices for internal Chinese consumption are doctored to allow the government to spy on the people operating the devices if required. Ditto the hardware produced in China.
And to me, this illustrates the near-futility of believing that digital regs, like GDPR in Europe etc, are going to be even remotely effective. Because I don’t think they are, no matter how many laws the EU and other governments pass. My point is, show me it is possible to top-shelf online-porn without creating a 24/7 big-brother dystopia, and I will believe cyberspace can be regulated by traditional political structures, otherwise there is no basis for such a belief.
There are good actors and bad actors in every walk of life. It doesn’t follow automatically that all powerful men are bad. We do know of some corrupt, evil personalities (Weinstein, Epstein, Saville, Burmese Generals), but it’s not so crazy that they are all like this!!
Mercurial, lasting changes have arrived, wether we like it or not. Some good, some bad, some wonderful and some terrible. History has always been like this …..
In the midst of this complicated, dysfunctional mess (still in it’s infancy), it would probably be wise, to be thankful that global warming is now at least, being taken seriously.
If we stand uncompromisingly against a mighty wave, it will wash us away. When the wind of change blows, the wise investor builds windmills.
Your list of bad guys is pretty brief – I would add Biden, Soros, Bezos, Zuckerberg, Gates, Dorsy, Blair, and everyone who is a Billionaire off finance.
How do you know what ‘seems to bother’ any one else? You used to be one of the good guys, don’t start spreading false narratives about people being relaxed about Blair and Gates running their lives. If you think facial recognition technology is the dreadful, authoritarian, chilling thing you say you do, write about that. Don’t write a pretend story about what is inside other people’s heads, which you cannot possibly know. It comes across as a fantasy and an attempt to persuade your readers to join the masses and be relaxed about the social credit score and ‘freedom passport’, rather than a criticism of the wicked system.
To be honest, I didn’t read it like that… I think he’s saying that most of the population don’t seem concerned at all, but they d*mn well ought to be. Personally I’m horrified by it, but most people swallow the “if you’ve got nothing to hide you’ve got nothing to fear” argument that tyrants always use to justify mass surveillance and control.
I stop at traffic lights, it doesn’t mean I don’t think roundabouts are a better approach to dealing with traffic converging from different directions. Obedience to the law is not the same as believing the law is intelligent. We are bullied into compliance with the state’s authoritarianism, we are quiet, private people. That doesn’t mean we accept it as lawful and that we don’t care that our freedom is being eroded.
I know what Timandra Harness (I think female, does programmes on R4 from time to time, used to write for Spiked) was trying to express, but her argument phrased in the way it is is dangerous, even if unintentionally.
I think the point that was being made is that you can deduce whats inside other peoples head by observing their behaviour. Millions of Britons have Facebook accounts, and almost every-one uses WhatsApp (I’m expecting the replies of ‘well I don’t’), people give away their data for no obvious benefit – without even thinking about it. Not giving away your data is actually pretty easy, but how many peole do you think care enough to:
Once you start thinking about where you are leaking this data, its pretty easy to stop – but most people simply don’t care even when you tell them its happening.
PS: I’m not paranoid, It really is just as easy to use fake details as real ones. While many people would recognise me as Bertie – its not ever going to appear on any offical paperwork – and I wasn’t born precisely on the Unix epoch
I do pretty much all the things on your list. I’ve been writing computer systems for over 40 years, and I understand how and why to do it – as your epoch reference suggests that you do. Also, my age makes it easier to live on the periphery of most of this tech, It won’t be long before it’s nigh impossible to do. There’s an intersting article / discussion on the Linux Weekly News website about IoT and how hard it’s becoming to escape.
Tracked down the link (wow thats an aweful looking website)
https://lwn.net/Articles/850218/
One thing to say about IoT phoning home is that it wouldn’t necassarily have access to any specific data about the user of the device. From the mobile connectivity it could work out an approximate location but it would be very hard to tie a fridge to a TV to a household – even if they were both from the same company – unless you were silly enough to register them with the supplier. As a side note – I watch almost all of my TV via a laptop plugged into it – just because the laptop has Ad-blockers.
There’s a link somewhere to a Bruce Schneier post where he notes that you can’t buy a new car these days that’s not connected to the internet – difficult not to give your details to the dealership / manufacturer / Insurance company / DVLA etc.
yes it is an awful web site, not changed in 20 years, but it’s a good source for technical info
Yes, people give away their data freely. Any time a service is provided to you for free, it means that you are the product, not the consumer. But this contract never assumed that the data collected would be used to harm us and control us. No one assumed that the data would be the pretext for determining who is or is not worthy of taking part in the numerous benign daily transactions that mark life in a free society. The data is on the verge of being collated into a giant permission slip that authorities will use in order to grant privileges that we used to call freedoms. In that sense, our initial bargain with big tech has changed dramatically.
Out of interest what do you consider to be better than Google? For any “controversial” topic I use Startpage or Qwant. I find Google generally has better results for things without an ideological bent to them, though.
I use Duck Duck Go, but it is a poor search engine, but good enough. I really should get Linux I guess, but cannot be bothered.
Just look at the Writer’s bio, BBC Presenter, which is why racism is front and center, then welfare and criminals being treated differently, And the very name of her Book – ‘Big Data, Does Size Matter?’ is both childish and puerile, and gets some reference to sex in (and a dig at men), BBC has its own stamp the simplest algorithm could spot in a second. I guess these are the product of modern education systems, like she says, the Post Code, school, university, courses taken, and any simple algorithm could say if you are BBC fodder.
‘wrongly tagged with a poor credit history or tendencies towards political extremism.’ Well I have a perfect credit score, I always groomed my credit score because I used to rely on large personal loans to do my work (house building) But then I know I am on all sorts of political databases too, I do know how I Always have to go through the bomb sniffer when I fly (they take me and about 2 others into a room where sticky tape is rubbed on your collar, waist, shoes, hands, carry on bag inside and out, then it is fed into what must be a mass spectrometer to see if you have explosive residue. I ask them why me and they say it is just random, but every time shows that is not the case. I also have other things happen, so I know I am on databases. But then looking at my past I can see things which would incline to be on one as each is nothing, but mega data compiles them – and I have been extreme on line since my first, 5 1/2 inch floppy, DOS operated, (made me love Microsoft when they did windows with a Mouse instead of using keyboards to control the computer like DOS) Amstrad computer – and been to very weird places, and off the map and grid for years at a time, and who knows what else.
I always use a VPN, Never fail to wipe my history and data every time I close a page, use aliases, one even has its own credit card, which I have had years, or new ones, Never owned a cell phone, never used vehicles which have GPS, but then I have been finger printed a lot, and photographed, Never let any device know my passwords, or my history or preferences, or even name, and I suspect it is all for nothing, that the system is way beyond that. We are bugs pinned to a board behind glass, labeled and identified.
Also – China has YOU totally tracked and a social credit score built. They have a mania for snooping, and so data mine everything on every human on the planet – they never know which guy will end up in charge of mineral rights in Tanzania, or who might say bad things about them, but mostly because of their snooping OCD.
There is a constituency of people who will obsess more about the fact their neighbour has a bought a new car than the right to protest.
They don’t think these issues will affect them, or at least won’t affect them in a negative way.
They view the world through their own experience and until a problem comes to their doorstep they will shrug their shoulders.
By the way those are the very people who created The problems at the first instance and they are exploiting natural resources, destroying nature and humanity, behaving as the planet belongs to them only.. whereas they make everyone guilty because please think before you print but the printer’s manufactures’ have never had the slightest thoughts.. It could not be a better time, they are hold accountable for all their crimes.. I have never asked for cars, internet, Tv, satellites.. who has? who cares? I have ditched Tv since many many years ago.. I can lead a much healthier life without Tv, mobiles, internet, cars.. life has been better and far more creative without them for a very very long time.. But what they mean is that: we will own nothing and be happy.. and that is the meaning of corona to give away the private, personal body.. I am in for less less less technology, medicine, world organizations, puppet governments and mostly, it is the time the criminals who pointing their finger to us, to pay for their crimes..it is only fair..
I doubt that the British state has the competence to create a Chinese style surveillance system. Our future is more likely increasing anarchy and low level civil war as the discrepancy between the promises of democracy and it’s ability to deliver becomes ever more glaring.
They could contract it to Gates. Easy, and cheap.
Used a Microsoft device any time recently?
Schwab sold his soul to the devil to get were he is, like,Soros, Zuckerberg, Dorsey, Gates, and Bezos. This means what they tell you is not in your best interest.
Can I suggest that actually we want facial recognition technology to work well in all situations, including walking past the metropolitan police FRT van?
If it is well trained, on a decent number of samples, the number of false positives and negatives (ie where it gets identification wrong) will be low. That means I won’t be confused with that criminal on the TV last night, and it means the technology can focus on actually identifying those known to the security services as a threat.
Your naivety is touching Bill and quite possibly the answer to the article’s question of how so many of us allow this technology to take over every aspect of our lives so easily. I hope none of the following should befall you as a result:
Mortgage/life insurance/health insurance application rejected – No explanation given
Driving licence/GHIC card application rejected – No explanation given
Airline booking rejected – No explanation given
Job application rejected – No explanation given
FRT is the missing link between the databases and algorithms that the public and private bodies which issue the above “privileges” to us use to assess our worthiness for approval. This is dystopian present, not future. We live in a country where anything we have ever said, done or written can be recorded by the Police as a “non-crime hate incident” without our knowledge and may disqualify us from any job requiring an enhanced DBS check.
Here is a quote from an article in Wired magazine from last month:
“For the last two years police and internet companies across the UK have been quietly building and testing surveillance technology that could log and store the web browsing of every single person in the country”.
The complete internet connection and browsing history of thousands of UK citizens is currently being collected and analysed under the 2016 “Snooper’s Charter” bill. There has been no official explanation of how this data will be analysed or stored.
For the moral, model citizens of Islington who conform to the Social Justice mantra in everything they say, do and think, cast your mind back. That website you used to research Extinction Rebellion, or the time you liked the “joke” Tweet calling for the stoning of Tory politicians, or the comment you made in the pub about how useful offshore trusts can be.
Be afraid, be very afraid because they’re looking at you too.
Paranoia, anyone?
Also, did anyone notice how the article’s author vouches for the value of trust between humans, only to selectively arbitrate that the (obviously human) actor implementing these technologies does not qualify for such trust?
Last but not least, we don’t live in China. If your paranoid scenario of “mortgage application denied” ever materialize, there are courts of law which very purpose is to afford you an avenue for defense.
Facial recognition software has obvious teething problems, but those can be solved. And if the paranoids were really considering society as a whole, someone would have at least mentioned all the good that can very obviously be derived from a more effective tool for police work.
So let’s all put on our big boy pants, acknowledge that any new technology also brings risk of misuse, but not to the point that we should panic and reject it outright. Consider that this paranoid mindset would have prevented every single technological advance that we enjoy today.
Were I describing a dystopian future your accusation of paranoia would be fair comment. I am talking about the UK as it is today. To address the points you raise:
Your mortgage application/ bank account/ life insurance application can already be refused without reason as they are granted by private companies. The only redress in law would be based on proof of discrimination under a protected characteristic such as race. As an example, an increasing number of UK citizens are having their bank accounts closed with no reason given (see Guardian story entitled “NatWest closed my account with no explanation” as just one example.
You say “we don’t live in China”. I would add agree but add the word “yet”. The only real difference is that we don’t yet have a social credit system, mainly because China have had longer to develop their FRT infrastructure.
I understand the mindset of “all is paranoia until it happens to me”. Similarly it is a perfectly understandable position to believe that government and private companies’ use of one’s data will only ever be benign for “good” people like oneself.
You may be right, you may be wrong but someone else is rolling the dice on your behalf. Your trust in them is admirable.
Out of curiosity, Stephen: You do have more than one bank in the UK, right? Just checking…
IN CAPS SO YOU SHEEP CAN HEAR – THE POLICE ARE BEING DEFUNDED AND MADE USELESS TO INCREASE CRIME!!!!!! THIS IS EXACTLY TO MAKE YOU SHEEPLE ACCEPT THE POLICE STATE. LET THE CRIMINALS HARM YOU OR ONE YOU KNOW, THEN YOU WILL CALL FOR ABSOLUTE SURVEILLANCE.
With the current trial in Minnessota its a wonder anyone in America wants to join the police. If there were no police nothing stopping an area paying for its own private security-after all before 18th century ( start present day police ) life wasn’t that dangerous.
When I think of technology that is meant to help us I always think of a Jacques Tati film ( think it is Playtime) where Monsieur Hulot interacts, less than successfully , with the moden world of inventions. I would always be worried that facial recognition wouldn’t recognize me and wouldn’t let me out of the house , have my money or various other things-and your only answer is to phone an automatic call centre-we value your custom, your number is on hold , you are 410 in the queue
‘I would always be worried that facial recognition wouldn’t recognize me” I sometimes have this sort of worry, like is gravity going to reverse and I be shot into space, then what will I do?
Without gravity we are all in trouble-fortunately it is one of the few things equal to all mankind-even multi-billionaires can’t buy themselves an extra load of gravity
My thoughts, exactly. Thank you.
The problem is I hate criminals and want them caught, but unfourtnately, in my past I could have been caught for things, and I am glad I was not.