Getty
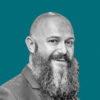
When I was first prescribed a statin to control my liver’s production of cholesterol, it required a (very good-natured) verbal tussle with my primary care physician. “You have a very healthy lifestyle,” he told me; “You are not at risk of developing heart disease in the next ten years.” I don’t care, I told him. My father died of heart disease, as did my maternal grandfather. Too young, both of them, they died too young.
The physician agreed (and wrote the prescription). So why did he begin with mild reluctance? Not an innate desire to avoid pharmacological interventions (else he would not have written the prescription.)
The conflict arose from a clash of algorithms. I had taken a statistical model to the surgery with me — a very informal one, but a model nonetheless; “Given the input of my family history, I believe my innate long-term probability of heart disease to be high, and wish to minimise that risk.”
Physicians tend not to think in terms of statistical model but the machines which sit on their desktops do. Dr P typed in various numbers (inputs) to describe me, which included family history, and various blood tests, and metabolic measurements, and lifestyle indicators… and the classification algorithm in his surgery’s software returned: “Medium risk.” The computer, in other words, said no.
On this occasion, and with this algorithm, the human discussants over-ruled its decision but with the rise of machine intelligence — a fancy-sounding name for “classification or prediction algorithms built on huge amounts of data” (to which Peter Franklin gives a very good introduction here) — the potential for conflict between the human users of the systems and the system itself, increases. And it’s not clear whose rights will take precedence.
Should you qualify for parole? An algorithm might determine that.
Should you be allowed to board an aircraft? An algorithm will determine that.
How should you drive from A to B?
Which irritating adverts will decorate your internet browsing experience?
Which film does Netflix suggest you watch next?
What insurance deal will the Halifax offer you on home contents cover?
Is it worthwhile spending money on a drug to prolong your life? An algorithm, increasingly, determines all of these.
Join the discussion
Join like minded readers that support our journalism by becoming a paid subscriber
To join the discussion in the comments, become a paid subscriber.
Join like minded readers that support our journalism, read unlimited articles and enjoy other subscriber-only benefits.
Subscribe