But what about all those false positives? Credit: Christopher Furlong/Getty Images
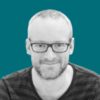
In ancient Greek mythology, Cassandra was cursed by the gods: she was given the gift of prophecy, but although her prophecies would always be true, no one would believe them. She foresaw that Helen’s abduction would lead to the destruction of Troy; she warned of the wooden horse, filled with Greeks, which was the instrument of that destruction. But her warnings went unheeded.
I don’t want to say I’m exactly like Cassandra. But I do want to say that everyone is ranting and raving about false positive rates at the moment, almost as if they didn’t listen when I was ranting and raving about them back in early April. It all seems to stem back to an article on Toby Young’s “Lockdown Sceptics” site a few days ago, in which the author correctly pointed out that if a test has a 1% false positive rate, that doesn’t mean that only 1% of positives are false.
From there it’s sort of spiralled out of control, with, among other people, Julia Hartley-Brewer saying that “the Government is planning to lockdown our country again when there is no evidence of a second wave” and John Redwood demanding that “government advisers today need to tell us how they are going to stop false test results distorting the figures”.
Here’s what’s going on. First, it’s absolutely true that the “false positive rate” does not on its own tell you the percentage of positive results which are false. Imagine this: you’ve got 10,000 people; you have a test that gives you the correct answer 99% of the time (i.e. 99% of people who do have the disease get a positive result, and 99% of people who don’t have the disease get a negative result); and you have a disease that only 0.1% of the population has (which is roughly what the ONS reckons is the real prevalence of Covid in the population).
So out of the 10,000 people, there are 10 people with the disease. Your test will probably tell you, correctly, that they all have the disease. But it will also give false positives to 1% of the 9,990 people who don’t have the disease. So it will probably tell about 100 entirely healthy people that they have Covid-19. So in this situation, given that a disease that only 0.1% of people have, a positive result on a test with a 1% false positive rate means you only have about a 9% chance of actually having the disease.
This is all very straightforward and exactly what Bayes’ theorem tells us. If you get a positive test, your chance of actually having Covid is dependent not just on how accurate (or technically how “specific”, the technical term for how good it is at avoiding false positives) it is, but also how common the disease is in the population.
What Hartley-Brewer, John Redwood and others are suggesting is that this means that, actually, there’s no real reason to believe that there is a second wave, because the positive test results hugely overstate the real number of cases. I think they’re wrong about that, and quite crucially wrong. Let me try to explain why.
Join the discussion
Join like minded readers that support our journalism by becoming a paid subscriber
To join the discussion in the comments, become a paid subscriber.
Join like minded readers that support our journalism, read unlimited articles and enjoy other subscriber-only benefits.
Subscribe