(Photo by Fox Photos/Getty Images)
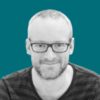
Imagine that you’re trying to decide which school you want to send your child to. Of course, your little darling is the most gifted and brilliant child in the world — anyone can see that! That time he set the headteacher’s hair on fire was only because he wasn’t feeling sufficiently challenged. Anyway, it’s time to find somewhere that will really push him. So you’re looking at the exam results of the various schools in your area.
Most of the schools report that 80% or so of their children achieve A-to-C grades in all their exams. But one school reports 100%. They all appear to be demographically similar, so you assume, reasonably enough, that the teaching is much better in that one school, and so you send little Mephiston there.
But a year later, his grades have not improved, and he is once again in trouble for dissecting a live cat in biology class. You dig a little deeper into the exam results, and someone tells you that the school has a trick. When a child doesn’t get a result between A and C, the school simply doesn’t tell anyone! In their reports, they only mention the children who get good grades. And that makes the results look much better.
Presumably, you would not feel that this is a reasonable thing to do.
It is, however, exactly what goes on a lot in actual science. Imagine you do a study into the efficacy of some drug, say a new antidepressant. Studies are naturally uncertain — there are lots of reasons that someone might get better or not get better from complex conditions like depression, so even in big, well-conducted trials the results will not perfectly align with reality. The study may find that the drug is slightly more effective than it really is, or slightly less; it may even say that an effective drug doesn’t work, or that an ineffective one does. It’s just the luck of the draw to some degree.
That’s why — as I’ve discussed before — you can’t rely on any single study. Instead, the real gold standard of science is the meta-analysis: you take all the best relevant studies on a subject, combine their data, and see what the average finding is. Some studies will overestimate an effect, some will underestimate it, but if the studies are all fair and all reported accurately, then their findings should cluster around the true figure. It’s like when you get people to guess the number of jelly beans in a jar: some people will guess high, some low, but unless there’s some reason that people are systematically guessing high or low, it should average out.
But what if there is such a reason? What if — analogous to the school example above — the studies that didn’t find a result just weren’t ever mentioned? Then the meta-analyses would, of course, systematically find that drugs were more effective than they are.
Join the discussion
Join like minded readers that support our journalism by becoming a paid subscriber
To join the discussion in the comments, become a paid subscriber.
Join like minded readers that support our journalism, read unlimited articles and enjoy other subscriber-only benefits.
SubscribeMm, a good article, the precepts of which can be applied much more widely that just to drug trial data.
The whole climate change and global warming agenda, now so widely accepted without question, is largely based on just such manipulation of scientific studies, rejection of data which do not support the hypothesis, and splicing together different sources of data where this statistically invalid process does support it.
For those who want the whole story, Andew Montford’s detailed treatise ‘The Hockey Stick Illusion’ tells it.