Credit: Jens Schlueter/Getty Images
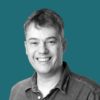
Today’s UnPacked features a story that should have a got a lot more attention than it did.
Covered late last year by Devin Coldewey of the TechCrunch website, it concerns a type of AI system called a generative adversarial network or GAN.
A GAN consists of two halves: a ‘discriminator’ and a ‘generator’. By having labelled items of data fed to it, the discriminator learns to identify the patterns that correspond to the labels – so that it can go on to identify similar patterns in unlabelled data. An example would be a system set up to identify particular faces in CCTV footage.
The generator works the other way round – it generates or modifies data to create simulated patterns with the objective of convincing an observer that they correspond to a particular label. A rather sinister example might be the simulation of CCTV footage containing recognisable faces. Feedback from the observer enables the system to learn to produce evermore convincing fakes.
By pitching its generator and discriminator components against one another, a GAN computerises the feedback process, thus greatly speeding up the rate of learning.
The TechCrunch story concerns an experiment by Stanford and Google researchers, in which a GAN was given the task of converting aerial photography into the sort of image you might see on a sat nav app:
Join the discussion
Join like minded readers that support our journalism by becoming a paid subscriber
To join the discussion in the comments, become a paid subscriber.
Join like minded readers that support our journalism, read unlimited articles and enjoy other subscriber-only benefits.
Subscribe