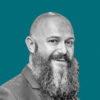
Truth lies in fiction is the pleasing paradoxical assertion that justifies my avoidance of dreary books about politics, or those suddenly-fashionable works about how to think better. But truth doesn’t exist only between the pages of a well-written novel. Its shadowy form can sometimes be discerned flickering on the walls of the cave inhabited by the experimental sciences.
Consider this very modern problem: I have given a test drug to 20 patients, and 15 of them responded positively. That’s a 75% ‘response rate’, in the jargon, in this sample of patients.
But common sense will tell you that 75% isn’t the drug’s true response rate (would you expect exactly 15 patients to respond out of every further 20 who were exposed to the treatment? Or sometimes 14, sometimes 18, and so on?) So: the observed response rate isn’t the truth. But – and again, applying merely to common sense, and with the supposition that I didn’t rig the trial in some way – surely a response rate of 75% is more probably the true response rate than, say, 25%?
To peer at the truth about a theory concerning the world (in this case, the true response rate of the drug) usually requires an act of inductive inference: from experimental observation (“We observed a 75% response rate in this study”), to statement about the truth (“The true response rate is more likely to lie between 50% and 90% than it is to lie below 10%”). You’ll never know the truth (shades of Jack Nicholson) – you can’t expose every possible patient throughout the Earth’s history to the drug, and count how many of that infinite set would respond – yet you can still make valid claims about it. Roll that thought about your head a moment, and then tell me Statistics isn’t sexy.
That there exists an inductive logic that more or less works is thanks in part to the Reverend Thomas Bayes, whose simple inquiry in the 18th century provides the answer to what I called a “very modern problem”.
Here’s his version, posthumously published by his friend Richard Price, in 1763:
Other than f-for-s, this is essentially the “what is the probability that the true response rate for this drug lies between 50% and 90%, given that we’ve observed a 75% response in this trial?” question with which we opened.
Join the discussion
Join like minded readers that support our journalism by becoming a paid subscriber
To join the discussion in the comments, become a paid subscriber.
Join like minded readers that support our journalism, read unlimited articles and enjoy other subscriber-only benefits.
SubscribeGraeme – only just read this. An excellent read. I did Stage 1 statistics about 25 years ago – and loved it. Even introductory level competency has been a huge help in thinking about complex issues. But also a frustration as I know enough to know that many people mangle and misuse statistical data, but usually not enough to coherently point it out. Oh well….