A Virtual Reality game. Credit: David McNew / Getty
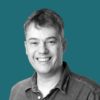
Around this time last year the AlphaZero AI system taught itself how to play chess in just four hours. It then thrashed the best chess player in the world (itself a computer program) over the course of a hundred games.
As a demonstration of the power of machine learning, AlphaZero (and similar programs) caused some alarm – see, for instance, the Shadow Foreign Secretary’s take on the issue for UnHerd. If a machine mind can become a chess grandmaster between breakfast and lunch, then what might it achieve by dinner-time, or next week or, gulp, next year?
Well, here we are twelve months on, and human beings are still in charge of the planet. Whatever AlphaZero is up to these days, it isn’t ushering in the Singularity.
In a short post for MIT Technology Review, Karen Hao explains why game-playing AI systems are limited in what they can achieve:
“[Reinforcement learning] is a category of machine learning techniques that uses rewards and penalties to achieve a desired goal. But the benchmark tasks used to measure how RL algorithms are performing—like Atari video games and simulation environments—don’t reflect the complexity of the natural world.
“As a result, the algorithms have grown more sophisticated without confronting real world problems—leaving them too fragile to operate beyond deterministic and narrowly defined environments.”
A game like chess has clear rules and defined objectives. Furthermore, the success, or otherwise, of any sequence of moves can be assessed in terms of interim and final game outcomes. This means an AI chess player can generate vast quantities of labelled data by playing games against itself – in which winning patterns of moves can be recognised and ‘learned’ in a process of trial and error.
Tasks in the real world, however, are not like a game. Even if an objective is clearly defined, the rules for achieving it may be neither obvious nor unchanging. Through trial and error, an AI system may be able to work out what the rules are (or at least a rudimentary version of the rules), but to recognise right and wrong answers (and hence the patterns of rightness and wrongness from which rules can be derived) it usually needs an external source of labelled data.
Join the discussion
Join like minded readers that support our journalism by becoming a paid subscriber
To join the discussion in the comments, become a paid subscriber.
Join like minded readers that support our journalism, read unlimited articles and enjoy other subscriber-only benefits.
Subscribe