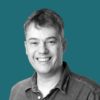
In a world where we have access to more information than any of us has time to verify, how do we decide what is both true and useful?
The answer, is that we make prior assumptions about what (and who) is trustworthy and relevant. Furthermore, we tend to do so collectively – subscribing to the shared assumptions of the groups we want to be part of.
Fair enough. We need those filters, otherwise we’d be overwhelmed. The problem though is when the filter become so restrictive that it only allows through the information and ideas that confirm existing assumptions. From that point, it ceases to be a defence and becomes a cage – a bubble within which different perspectives have no place and new understanding becomes impossible.
Such bubbles are typically constructed from linguistic components – facts, theories, jargon, slogans, arguments, talking points, memes, cultural references. However, the thickest bubbles are made out of numbers not words.
While words are open to interpretation, numbers are an expression of objective truth: 2 plus 2 equals 4 and nothing else. We may fear the dark arts of statistical manipulation, but what could be more objective than a direct, unfaked observation?
Unfortunately, there are pitfalls there too. The point is made in an eye-opening piece by Aaron E Carroll for the New York Times. It concerns that most wholesome of corporate activities – the workplace ‘wellness’ programme.
Join the discussion
Join like minded readers that support our journalism by becoming a paid subscriber
To join the discussion in the comments, become a paid subscriber.
Join like minded readers that support our journalism, read unlimited articles and enjoy other subscriber-only benefits.
Subscribe