Scientific data-mining is contaminating serious study
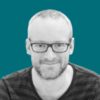
You may not have heard of the most surprising scientific result of all time. It was published in the journal Psychological Science1, and it found that listening to ‘When I’m 64’ by the Beatles literally made you younger by nearly 17 months.
Not “made you feel younger”, or “made your brain younger”: the study demonstrated that listening to certain songs made you younger. And these surprising findings met the standard of “statistical significance” which most scientific journals demand.
In fact, the three authors of the study had set out to deliberately make a point. They wanted to show that, by using routine statistical methods2, you could find apparently solid results that were not merely unlikely but patently absurd.
The problem is that the standard of “statistical significance” is too easily subverted. And to prove it, the three authors used tricks to find relevant-looking patterns out of pure, random fluke results. We might call their techniques “cheating”, but they are routinely used in almost all walks of science.
Say you’re running an experiment into whether or not a coin is biased towards heads. You flip the coin three times; it comes up heads three times. Does that prove your hypothesis? No: sometimes you get three heads in a row just by chance.
That’d be called a “false positive”. Your chance of getting three heads in a row on a fair coin is 1 in 8, or as a scientist would write it, p=0.125.
In 1925, the great statistician RA Fisher arbitrarily decided3 that “significance” should be defined as p=0.05, or a 1 in 20 chance of a false positive. That definition is the standard that most research is still held to. Your three heads in a row wouldn’t do it, but five would: that’d be a 1 in 32 chance, or p<0.03.
The “When I’m 64” study had p=0.04, and thus met the threshold.
To prove the thesis, they did a simple test: they took 20 subjects, randomly divided them into two groups, and then played one group the Beatles and one group a control song, “Kalimba” by Mr Scruff. In theory, randomly dividing the two group means that they should be, on average, the same; the only difference should be the intervention, which song they listened to. And that means that, in theory, if you find a significant difference between the two groups, it has to be caused by that intervention. It’s a randomised controlled trial, just the same as is used to test cancer drugs.
And the study found that the “When I’m 64” group was, on average, 1.4 years younger than the control group, and found that this difference was statistically significant. So they had proved – to the standard most journals demand – that “When I’m 64” causes you to get younger.

Essentially, they gave themselves lots of chances to get that 1 in 20 fluke, then hid all their failed attempts at getting five heads in a row.
For instance, as well as making their 20 participants listen to the Beatles and Mr Scruff, they also asked them to listen to “Hot Potato” by the Wiggles. And they also asked 11 other questions, including how old they felt, their political orientation, whether they referred to the past as “the good old days”, and so on.
This allowed them to look at lots of different possibilities which might demonstrate their conclusion. Did the people who listened to “Hot Potato” happen, by chance, to be more politically conservative? Did people who listened to the Beatles say “the good old days” more often? No? Then the scientists didn’t report on that. There were dozens of ways to analyse the data, easily enough to get a 1-in-20 result just by fluke.
This behaviour is called “hypothesising after results are known”, or HARKing4. It means waiting until you’ve got your data, then sifting through it in imaginative ways until you find something that looks like a result. It gives you a much higher chance of (falsely) reaching a “significant” finding: using tricks like this, collectively known as “p-hacking”, Simmons, Nelson and Simonsohn say that the chances of a false positive can rise above 60%.
And this happens all the time, in real papers. A study by the Oxford University Centre for Evidence-Based Medicine5 found that the top five medical journals in the world regularly publish studies which change what they’re measuring after the trial begins, a practice related to HARKing.
Chris Chambers6, a professor of psychology at Cardiff, tweeted recently that he’d had a paper rejected for having conclusions that were “not necessarily novel”.
Short thread. Had a paper accepted today at @royalsociety Open Sci & another rejected at Appetite.
Here’s some review comments from Appetite, not as a whinge (I’m too old & leathery to complain about reviews) but as a reminder of the challenge we face reforming the field /1
— Chris Chambers (@chrisdc77) January 11, 2018
“We could have been more novel,” he wrote. “We could have HARKed this paper up the wazoo or p-hacked it. We didn’t.”
The trouble is a deep-seated flaw in the process of “peer review”. “They judge the work,” Chambers said, “not just on how important the question is and how well the study is designed, but also whether the results are novel and exciting.”
This leads to a fundamental problem.When you select what to publish based on results, the knowledge base only reflects some of the truth. According to Chambers: “Positive studies get into journals but equally high-quality studies that don’t show results are basically censored.”
This sort of “publication bias” is well-documented 7. “Publication bias is catastrophic for medicine,” says Chambers. It also has profound implications for government policy: in the US, the federal government set up a $22 million (£16 million) programme called “Smarter Lunchrooms” on the basis of studies that contained flawed or missing data, several of which have since been retracted or corrected.
Luckily, there are people trying to do things about it, such as ensuring the preregistration of hypotheses, to prevent HARKing. Chambers is the founder of an initiative called Registered Reports: journals who sign up for it review a study on the basis of the study methods alone, and agree to publish regardless of the results.
This would take publication bias out of the equation and equally removes the incentives for researchers to data mine for significant findings, because the novelty or otherwise of the results is unimportant.
Such initiatives won’t make science perfect. But they almost certainly would improve things by aligning scientists’ incentives with those of society. They would stop fruitless research, prevent ineffective policy incentives and encourage straight-forward scientific innovation. Our lives would be made measurably better.
Join the discussion
Join like minded readers that support our journalism by becoming a paid subscriber
To join the discussion in the comments, become a paid subscriber.
Join like minded readers that support our journalism, read unlimited articles and enjoy other subscriber-only benefits.
SubscribeAt the first mention by some deranged trans ideologue that men can become women, and vice versa, it would be expected that every biologist would have joined together to deride the idea with a chorus of mockery. But they didn’t.
The vast majority of biologists have remained silent, (most likely through fear of repurcussions) and the general view of biologists has been left to be asserted by a loud and aggressive minority of careerist senior academics in influential positions. I doubt that there is anything more than a small minority of those senior academics who are true believers in the trans nonsense. But if they want to keep their positions, or to advance, they are obliged to go along with the DEI woke stupidity that is being imposed on all academic staff from the university management.
The reason that nonsense like trans ideology can now spread so quickly is that as a society, for decades, we have increasingly looked to academia as a objective, trustworthy source of data. When government decides policy, or people publicly debate various controversial issues such as immigration, crime or the validity of “gender identity”, they inevitably will use studies produced by academics as a basis to support or oppose a particular stance.
But now that it becomes more and more apparent everyday that the universities, professional associations and publications have been entirely captured by leftist ideologues (as the author’s experience evidences), to continue to have any trust whatsoever in any academic studies, data or conclusions, is absurd.
Ask why schools are allowed to teach this wretched trans nonsense to children, and a series of academic studies and guidance from professional bodies will be quoted supporting the idea that it is the best interests of children. For many people, and for government, the continued strength of the presumption that academics are unbiased truth seekers producing objective data, is enough to make them override their common sense.
We need an awakening among the public and government as to the extent of the capture of academia by “progressives”. It should be understood that any academic study, data and conclusions must be regarded with suspicion and automatically presumed to be contaminated by ideological bias. The moment some “progressive” starts to quote an academic study to support their position, tell them that given the abject corruption of academia by Leftists, it is of no evidential worth.
“I doubt that there is anything more than a small minority of those senior academics who are true believers in the trans nonsense.”
Not one single living individual on this planet with an IQ over about 90 sincerely believes in the trans nonsense.
Richard, sadly I am not sure you are completely right, for this reason: the vast majority of the true believers are victims of an *emotional*, not a *rational* impulse. They can have IQs above 150, but if they are determined to be thought virtuous, good people, or if they benefit either financially or socially (dinner parties become festivals of nodding at each other smugly), then they’ll jump on board so fast the boat nearly capsizes.
Being the one who sticks to principle or reason, when the currency of success or acceptance is agreeing with the cool kids, can be very very uncomfortable, as I learn regularly to my cost.
Foucault and Butler both write that the sexed body is created and recreated by each episteme. This is taught as philosophical critique in universities entailing that our episteme is one where gender identity supercedes sexed or even sexual identity.
Foucault and Butler both write that the sexed body is created and recreated by each episteme. This is taught as philosophical critique in universities entailing that our episteme is one where gender identity supercedes sexed or even sexual identity.
I’d love to hear from those trans nonsense sceptics
A hell of a lot of them lie about it then. Australia’s Julia Gillard being one and she (can i say that about her? – (ditto her)) is what I would define as a woman. Also PM in waiting Starmer, though he isn’t a woman no matter what he thinks.
PS Why do we never have headlines about ‘Trans Men’ making it into the National Team or breaking Men’s World records for anything physical? One might think Testosterone and puberty were important in determining something.
Quinn, a trans man, made it into the Canadian national team.
Quinn, a trans man, made it into the Canadian national team.
Richard, sadly I am not sure you are completely right, for this reason: the vast majority of the true believers are victims of an *emotional*, not a *rational* impulse. They can have IQs above 150, but if they are determined to be thought virtuous, good people, or if they benefit either financially or socially (dinner parties become festivals of nodding at each other smugly), then they’ll jump on board so fast the boat nearly capsizes.
Being the one who sticks to principle or reason, when the currency of success or acceptance is agreeing with the cool kids, can be very very uncomfortable, as I learn regularly to my cost.
I’d love to hear from those trans nonsense sceptics
A hell of a lot of them lie about it then. Australia’s Julia Gillard being one and she (can i say that about her? – (ditto her)) is what I would define as a woman. Also PM in waiting Starmer, though he isn’t a woman no matter what he thinks.
PS Why do we never have headlines about ‘Trans Men’ making it into the National Team or breaking Men’s World records for anything physical? One might think Testosterone and puberty were important in determining something.
I blame Brexit, those damn Brexiteers started this idea when they said “Don’t trust the experts!” AND now who does?
‘Caveat Emptor’ & ‘Follow the money.*’ Are probably the two most useful rules to hold in your head when reading anything.
Power = Money
“I doubt that there is anything more than a small minority of those senior academics who are true believers in the trans nonsense.”
Not one single living individual on this planet with an IQ over about 90 sincerely believes in the trans nonsense.
I blame Brexit, those damn Brexiteers started this idea when they said “Don’t trust the experts!” AND now who does?
‘Caveat Emptor’ & ‘Follow the money.*’ Are probably the two most useful rules to hold in your head when reading anything.
Power = Money
At the first mention by some deranged trans ideologue that men can become women, and vice versa, it would be expected that every biologist would have joined together to deride the idea with a chorus of mockery. But they didn’t.
The vast majority of biologists have remained silent, (most likely through fear of repurcussions) and the general view of biologists has been left to be asserted by a loud and aggressive minority of careerist senior academics in influential positions. I doubt that there is anything more than a small minority of those senior academics who are true believers in the trans nonsense. But if they want to keep their positions, or to advance, they are obliged to go along with the DEI woke stupidity that is being imposed on all academic staff from the university management.
The reason that nonsense like trans ideology can now spread so quickly is that as a society, for decades, we have increasingly looked to academia as a objective, trustworthy source of data. When government decides policy, or people publicly debate various controversial issues such as immigration, crime or the validity of “gender identity”, they inevitably will use studies produced by academics as a basis to support or oppose a particular stance.
But now that it becomes more and more apparent everyday that the universities, professional associations and publications have been entirely captured by leftist ideologues (as the author’s experience evidences), to continue to have any trust whatsoever in any academic studies, data or conclusions, is absurd.
Ask why schools are allowed to teach this wretched trans nonsense to children, and a series of academic studies and guidance from professional bodies will be quoted supporting the idea that it is the best interests of children. For many people, and for government, the continued strength of the presumption that academics are unbiased truth seekers producing objective data, is enough to make them override their common sense.
We need an awakening among the public and government as to the extent of the capture of academia by “progressives”. It should be understood that any academic study, data and conclusions must be regarded with suspicion and automatically presumed to be contaminated by ideological bias. The moment some “progressive” starts to quote an academic study to support their position, tell them that given the abject corruption of academia by Leftists, it is of no evidential worth.
Leftist ideologues are promoting feelings over facts because feelings and emotions can be manufactured, manipulated, directed, and controlled, whereas facts, evidence, and reason – based on the real world – are far more resilient. The result is a form of counter-Enlightenment; rather than evidence leading to hypotheses, theories, and eventually facts based on the strength and nature of that evidence, any facts which might go against the dominant ideological position must be suppressed. ‘Tolerant intolerance’ as the critical theorists declared.
Indeed. Being a woman is not a feeling, it is a fact. Putting on a dress may make someone feel more feminine but it doesn’t make him/her a woman or more of a woman. A woman might develop cervical cancer (it is a possibility), a man never will regardless of how much make up he wears, how feminine his attire, how much surgery he has. Similarly, a woman will never develop prostrate cancer. Being a woman is a biological fact.
That view is not confined to the left.
Indeed. Being a woman is not a feeling, it is a fact. Putting on a dress may make someone feel more feminine but it doesn’t make him/her a woman or more of a woman. A woman might develop cervical cancer (it is a possibility), a man never will regardless of how much make up he wears, how feminine his attire, how much surgery he has. Similarly, a woman will never develop prostrate cancer. Being a woman is a biological fact.
That view is not confined to the left.
Leftist ideologues are promoting feelings over facts because feelings and emotions can be manufactured, manipulated, directed, and controlled, whereas facts, evidence, and reason – based on the real world – are far more resilient. The result is a form of counter-Enlightenment; rather than evidence leading to hypotheses, theories, and eventually facts based on the strength and nature of that evidence, any facts which might go against the dominant ideological position must be suppressed. ‘Tolerant intolerance’ as the critical theorists declared.
I used to subscribe to Sciam. I cancelled my subscription several years ago when it started to break its links with enlightened thinking. I can only imagine its association with actual science has become even more tenuous in the intervening time.
I used to subscribe to Sciam. I cancelled my subscription several years ago when it started to break its links with enlightened thinking. I can only imagine its association with actual science has become even more tenuous in the intervening time.
As a parent, when my 12 year old daughter asks me if she can get a tattoo as soon as she turns 16 (she’s planning ahead) I tell her it’s my duty to protect the future her from the decisions of the childish her. Therefore I will refuse permission until she is an adult, as a tattoo is too permanent a decision. If she asked for a gender transition, with the associated hormone treatments, I would refuse for the same reasons. Unfortunately from what I understand she probably wouldn’t need my permission and my protection would be bypassed.
As a parent, when my 12 year old daughter asks me if she can get a tattoo as soon as she turns 16 (she’s planning ahead) I tell her it’s my duty to protect the future her from the decisions of the childish her. Therefore I will refuse permission until she is an adult, as a tattoo is too permanent a decision. If she asked for a gender transition, with the associated hormone treatments, I would refuse for the same reasons. Unfortunately from what I understand she probably wouldn’t need my permission and my protection would be bypassed.
Conversion therapy – indoctrination. How do they differ? It seems to me whilst busily indoctrinating society, trans activists are campaigning against conversion therapy. Surely the trans activists are working extremely hard on the indoctrination front to convert the youth of the country to their ideology.
Aren’t the opponents of what they call “conversion therapy” engaging in something very similar to what they claim to be the case with “conversion therapy”?
They are not doing it alone they are being funded and encouraged. The gender agenda is very much a part of the UN’s Agenda 2030.
Aren’t the opponents of what they call “conversion therapy” engaging in something very similar to what they claim to be the case with “conversion therapy”?
They are not doing it alone they are being funded and encouraged. The gender agenda is very much a part of the UN’s Agenda 2030.
Conversion therapy – indoctrination. How do they differ? It seems to me whilst busily indoctrinating society, trans activists are campaigning against conversion therapy. Surely the trans activists are working extremely hard on the indoctrination front to convert the youth of the country to their ideology.
There is an increasing and unsettling rise in the sexualisation of children mainly via LGBTQetc, the trans movement and various left wing media (the Guardian thinks the recent movie Sound if Freedom about child trafficking is extreme right wing propaganda and QAnon aligned).
In my view these people want to have sex with children, why else the sexualisation of children.
I’m afraid that if J Michael Bailey is not in to the idea that it’s ok to have sex with children any subsequent writings he submits for publishing is going to be either refused or vilified.
I suspect that a wide range of influential sexual deviants (for want of a better term) resent the general population’s resistance to their sexual deviations (sometimes tagged as “preferences”). They probably hope that exposing children to those deviations at a early age will destroy any natural aversion they might have and a whole generation will grow up completely uncritical of any sexual deviation however bizarre. A Queered utopia, I guess.
Why are key figures in our education authorities are allowing this to happen?
Because of their education experience?
Because of their education experience?
“(the Guardian thinks the recent movie Sound if Freedom about child trafficking is extreme right wing propaganda and QAnon aligned).”
Yes. It plainly is.
A sexual deviant writes…
..
Look. Shampoo Fashist. Some people think that you’re a satirist. If so, can you at least try to be amusing? This is just Nish Kumar-level inanity.
In what way? You don’t know, do you?
Don’t feed the trolls.
Don’t feed the trolls.
Wait, not wanting children to be sex-trafficked is ‘right-wing’?
Have you seen the movie or do you just read the reviews?
A sexual deviant writes…
..
Look. Shampoo Fashist. Some people think that you’re a satirist. If so, can you at least try to be amusing? This is just Nish Kumar-level inanity.
In what way? You don’t know, do you?
Wait, not wanting children to be sex-trafficked is ‘right-wing’?
Have you seen the movie or do you just read the reviews?
Not new news
https://www.bbc.co.uk/news/magazine-26352378
I suspect that a wide range of influential sexual deviants (for want of a better term) resent the general population’s resistance to their sexual deviations (sometimes tagged as “preferences”). They probably hope that exposing children to those deviations at a early age will destroy any natural aversion they might have and a whole generation will grow up completely uncritical of any sexual deviation however bizarre. A Queered utopia, I guess.
Why are key figures in our education authorities are allowing this to happen?
“(the Guardian thinks the recent movie Sound if Freedom about child trafficking is extreme right wing propaganda and QAnon aligned).”
Yes. It plainly is.
Not new news
https://www.bbc.co.uk/news/magazine-26352378
There is an increasing and unsettling rise in the sexualisation of children mainly via LGBTQetc, the trans movement and various left wing media (the Guardian thinks the recent movie Sound if Freedom about child trafficking is extreme right wing propaganda and QAnon aligned).
In my view these people want to have sex with children, why else the sexualisation of children.
I’m afraid that if J Michael Bailey is not in to the idea that it’s ok to have sex with children any subsequent writings he submits for publishing is going to be either refused or vilified.
Interesting to see that the three links in paragraph six all connect to articles by female journalists – and all doing their bit to subvert the patriarchy.
Sex Redefined: The Idea of 2 Sexes Is Overly Simplistic by Claire Ainsworth: science writer with a PhD in developmental biology
Modern Mathematics Confronts Its White, Patriarchal Past by Rachel Crowell: writer on science and mathematics
Denial of Evolution Is a Form of White Supremacy by Allison Hopper: filmmaker and designer
Another Scientific American piece ridiculed by Michael Shermer in the “progressive ideology” link bears the unwieldy title:
Brief descriptions of the five authors (3 of whom are women) are included at the end of the lengthy opinion piece. All seem to be stronger on political activism than science.
Always good to know a bit about the actual people helping to make these institutions as woke as possible. After all, cancel culture warriors have no qualms about singling out those whose views they oppose.
As Alexander Adams revealed in his 2019 book Culture War the worlds of Art, Publishing and Mass Media are increasingly dominated by women – particularly at the level of administration (where the real power lies).
Everything in the western ‘democracies’ is increasingly feminised and racialised. The publications listed above reflect this.
Could it be all that Oestrogen water pollution?
Maybe the rather scary suggestions we’ll end up being the undefinable sex is right?
Mind you I wait with interest the rash of Sex Abuse trials that are eventually going to arise out of the encouraged behaviours of these ideologues. Savile’s escapades will be as nothing once the current Elite’s monopoly on power is broken, and I suspect that might not be as far away as I feared.
Could it be all that Oestrogen water pollution?
Maybe the rather scary suggestions we’ll end up being the undefinable sex is right?
Mind you I wait with interest the rash of Sex Abuse trials that are eventually going to arise out of the encouraged behaviours of these ideologues. Savile’s escapades will be as nothing once the current Elite’s monopoly on power is broken, and I suspect that might not be as far away as I feared.
fascinating observations – more evidence that trans is an intra-female issue and has very little to do with males
It is all about males! It’s driven by autogynephilic males and the children are simply the cover for them.
Trans is to a large extent a men’s rights movement. And very misogynistic.
I think there is some merit to your comment but to be fair trans could have never gained traction without the feminist revolution claiming that men and women are equal. Encouraging women to compete in the sexual and career marketplace with men – like men – surely threw open the Overton window on sex differences and what they are and mean? Boys Scouts being forced to enlist girls was surely the gateway to this and the list goes on.
I think there is some merit to your comment but to be fair trans could have never gained traction without the feminist revolution claiming that men and women are equal. Encouraging women to compete in the sexual and career marketplace with men – like men – surely threw open the Overton window on sex differences and what they are and mean? Boys Scouts being forced to enlist girls was surely the gateway to this and the list goes on.
Trans is to a large extent a men’s rights movement. And very misogynistic.
It has everything to do with males: pretend women (i.e. males) are driving transactivism into ever more delusionary territory.
The irony is ideologically left females seem to be great supporters of it, and abusive, possibly right wing, males, the greatest beneficiaries of it. Reminds me of this little and surprisingly still available piece from the BBC
https://www.bbc.co.uk/news/magazine-26352378
It is all about males! It’s driven by autogynephilic males and the children are simply the cover for them.
It has everything to do with males: pretend women (i.e. males) are driving transactivism into ever more delusionary territory.
The irony is ideologically left females seem to be great supporters of it, and abusive, possibly right wing, males, the greatest beneficiaries of it. Reminds me of this little and surprisingly still available piece from the BBC
https://www.bbc.co.uk/news/magazine-26352378
The over-production of academics is itself part of the problem because it becomes ever harder to attract attention and thereby get the funding needed to make a career. Reasonable propositions based on sound evidence just aren’t enough anymore.
Spot on. The publication of all these so called scientific studies seem to reflect the authors desperate need to write something, whether they believe it or not, to get recognised and quoted on their CV’s. Two books could be read by non scientists to start to see the major problems
‘Scientific feuds’ by Joel Levy and ‘ Science fictions’ by Stuart Richie.
Spot on. The publication of all these so called scientific studies seem to reflect the authors desperate need to write something, whether they believe it or not, to get recognised and quoted on their CV’s. Two books could be read by non scientists to start to see the major problems
‘Scientific feuds’ by Joel Levy and ‘ Science fictions’ by Stuart Richie.
Everything in the western ‘democracies’ is increasingly feminised and racialised. The publications listed above reflect this.
fascinating observations – more evidence that trans is an intra-female issue and has very little to do with males
The over-production of academics is itself part of the problem because it becomes ever harder to attract attention and thereby get the funding needed to make a career. Reasonable propositions based on sound evidence just aren’t enough anymore.
Interesting to see that the three links in paragraph six all connect to articles by female journalists – and all doing their bit to subvert the patriarchy.
Sex Redefined: The Idea of 2 Sexes Is Overly Simplistic by Claire Ainsworth: science writer with a PhD in developmental biology
Modern Mathematics Confronts Its White, Patriarchal Past by Rachel Crowell: writer on science and mathematics
Denial of Evolution Is a Form of White Supremacy by Allison Hopper: filmmaker and designer
Another Scientific American piece ridiculed by Michael Shermer in the “progressive ideology” link bears the unwieldy title:
Brief descriptions of the five authors (3 of whom are women) are included at the end of the lengthy opinion piece. All seem to be stronger on political activism than science.
Always good to know a bit about the actual people helping to make these institutions as woke as possible. After all, cancel culture warriors have no qualms about singling out those whose views they oppose.
As Alexander Adams revealed in his 2019 book Culture War the worlds of Art, Publishing and Mass Media are increasingly dominated by women – particularly at the level of administration (where the real power lies).
In the more than 30 years during which I have worked as a mental health professional, teacher, and researcher dealing with adolescents I have seen one fad after another flourish and then disappear. We have gone through periods in which every unhappy adolescent was diagnosed with borderline personality disorder, multiple personality disorder, eating disorders, ADHD, bipolar disorder, autism (“on the spectrum”), and so on. In each case, a trail of psychiatric casualties has been left in the wake of the fad du jour. However, this is the first such fad in which we have advocated calling a delusional belief ‘normal’ and then responding with poisoning, mutilation, and the disenfranchisement of parents. This fad will also pass, but it appears likely to leave a much larger list of casualties who are far more severely harmed than those who went through unnecessary and unproductive psychotherapy and exposure to unnecessary and often harmful psychotropic medications. We will one day look back on this phenomenon in shame, and the ‘activists’, ‘scholars’, and ‘gender affirming clinicians’ who benefitted from it will move on unscathed to join the next fad from which they can advance their careers, enrich themselves, and gratify their narcissism.
Sweden appears to have been ahead of the curve on this issue. We saw an exploding number of adolescent females reporting as trans. And we thought that the problem was that children were getting a false rosy picture of what it would be like to be trans, mostly out of social media. So they made a documentary in 3 parts called _The Trans Train_ and showed it on national television. You can watch it yourself on youtube (unless you are in Sweden in which case you need to get your copy from SVT).
There was an 65% decline in the number of referrals to gender clinics. Some of this may be due to other changes, but it was striking the number of children who weren’t aware, until the broadcast that most people who report gender dysphoria are homosexuals, not transsexuals, and that many people who undergo surgery have regrets. The notion that you can be gender non-conformant without being trans was another new piece of information for them.
see https://genderreport.ca/the-swedish-u-turn-on-gender-transitioning/ for a longer discussion in English and the youtube link.
Sweden appears to have been ahead of the curve on this issue. We saw an exploding number of adolescent females reporting as trans. And we thought that the problem was that children were getting a false rosy picture of what it would be like to be trans, mostly out of social media. So they made a documentary in 3 parts called _The Trans Train_ and showed it on national television. You can watch it yourself on youtube (unless you are in Sweden in which case you need to get your copy from SVT).
There was an 65% decline in the number of referrals to gender clinics. Some of this may be due to other changes, but it was striking the number of children who weren’t aware, until the broadcast that most people who report gender dysphoria are homosexuals, not transsexuals, and that many people who undergo surgery have regrets. The notion that you can be gender non-conformant without being trans was another new piece of information for them.
see https://genderreport.ca/the-swedish-u-turn-on-gender-transitioning/ for a longer discussion in English and the youtube link.
Bravo.
Bravo.
Perhaps it’s because so many of these people don’t have kids and therefore don’t understand how these things work.
Ten years ago it was tattoos, now it’s gender reassignment.
Perhaps it’s because so many of these people don’t have kids and therefore don’t understand how these things work.
Ten years ago it was tattoos, now it’s gender reassignment.
There was a heavily downvoted post from a pro-trans debater that sparked some interesting discussion. It has been removed. Could we please have it back?
A very worthwhile piece with an evenmore enlightening ‘pocket intervention’ below by the ever-enlightened trans lobby. They alone seem capable of defeating the Demiurge and bringing the (cis-gendered) world out of spiritual Darkness and into a single Light.
A very worthwhile piece with an evenmore enlightening ‘pocket intervention’ below by the ever-enlightened trans lobby. They alone seem capable of defeating the Demiurge and bringing the (cis-gendered) world out of spiritual Darkness and into a single Light.
Interesting, I remember reading Chesterton in my youth, half a century ago, never did I expect him to be as prescient as he was regarding belief.
Because you are a fraud duplicating the fraud of Litman.
The idea that children are not naïve, easily suggestible and can be made to believe complete nonsense, and that young people going through puberty are not heavily prone to confusion. peer pressure and fads, it a ridiculous denial of the reality that everyone of us has personal experience of.
Knowing what children and young people are like, and the enormous increase in the widespread distribution of indoctrinating trans ideology, the exponential rise in “trans identifying” children in exactly what we would expect to see.
Come now. Can you explain to us what the fraud is?
It seems that Michael Bailey has given an analysis of information from a large number of sceptical parents of transitioning children, and found some interesting conclusions. The interviewees are self-selected and quite likely biased, and there are no interviews with the children, but the article says so, explicitly, and discusses how this limits the conclusions one could draw. You may disagree with his hypothesis or his conclusions, but where is the fraud?
On the general situation, it seems to be established fact that the number of adolescents presenting themselves as trans has increased dramatically over the last decade, particularly for (natal) girls. Also that those who do so tend to have quite strong connections with people or internet sources that are pro-transition – which, in modern internet culture is not surprising. Well, duh! It seems highly unlikely that all of a sudden many more people are being ‘born in the wrong body’, so the increase in the number of transitioning children pretty much has to be caused by increased information, attention, and support for the phenomenon. That could mean either that the same number of children are inherently transgender and now find it more easy to express that. Or it could be that more children choose to identify as transgender as a social role that would improve their life situation, because this choice is now presented as more available and highly socially supported. Which explanation you prefer would depend on your a priori beliefs, there being no independent way of proving the real gender identity of anybody. More research on the circumstances might support one or the other theory (maybe you can link to some research that pushes in the other direction, so we can have a scientific look at this). Or you might choose to reject the search for evidence and decide based on prior beliefs, either that all these children really are transgender, or that many of them are likely not to be so. Whatever the underlying facts there could be social or political reasons for preferring one or the other approach.
But, again, where do you find the fraud in all this?
One small point, given my “a priori” beliefs, I say that the word “natal” is redundant, unless you are referring to South Africa.
I know you used brackets,.but still…
On the possibility that trans people come forward more readily now, the question would be, where were they holed up until the other day? There is plenty of examples of gender not conforming people, but trans people? (And don’t get me started on the suicide thing…)
I agree on the ‘natal’, but I am writing to Talia Perkins, who is clearly on the trans side of this particular chemical bond
. I do not want the discussion to go astray on the question of what I mean by ‘girls’.
Yes, it’s important to be clear. The term “natal female” simply ensures that we are all on the same page. The modifiier “natal” should be unneeded, but that’s not the current situation.
Yes, it’s important to be clear. The term “natal female” simply ensures that we are all on the same page. The modifiier “natal” should be unneeded, but that’s not the current situation.
I agree on the ‘natal’, but I am writing to Talia Perkins, who is clearly on the trans side of this particular chemical bond
. I do not want the discussion to go astray on the question of what I mean by ‘girls’.
One small point, given my “a priori” beliefs, I say that the word “natal” is redundant, unless you are referring to South Africa.
I know you used brackets,.but still…
On the possibility that trans people come forward more readily now, the question would be, where were they holed up until the other day? There is plenty of examples of gender not conforming people, but trans people? (And don’t get me started on the suicide thing…)
ROGD is a real phenomena. Trans is a delusion which is the hysteria of the time.
Those who promote this trans delusional psychosis are trying to groom more children to become deluded.
The idea that children are not naïve, easily suggestible and can be made to believe complete nonsense, and that young people going through puberty are not heavily prone to confusion. peer pressure and fads, it a ridiculous denial of the reality that everyone of us has personal experience of.
Knowing what children and young people are like, and the enormous increase in the widespread distribution of indoctrinating trans ideology, the exponential rise in “trans identifying” children in exactly what we would expect to see.
Come now. Can you explain to us what the fraud is?
It seems that Michael Bailey has given an analysis of information from a large number of sceptical parents of transitioning children, and found some interesting conclusions. The interviewees are self-selected and quite likely biased, and there are no interviews with the children, but the article says so, explicitly, and discusses how this limits the conclusions one could draw. You may disagree with his hypothesis or his conclusions, but where is the fraud?
On the general situation, it seems to be established fact that the number of adolescents presenting themselves as trans has increased dramatically over the last decade, particularly for (natal) girls. Also that those who do so tend to have quite strong connections with people or internet sources that are pro-transition – which, in modern internet culture is not surprising. Well, duh! It seems highly unlikely that all of a sudden many more people are being ‘born in the wrong body’, so the increase in the number of transitioning children pretty much has to be caused by increased information, attention, and support for the phenomenon. That could mean either that the same number of children are inherently transgender and now find it more easy to express that. Or it could be that more children choose to identify as transgender as a social role that would improve their life situation, because this choice is now presented as more available and highly socially supported. Which explanation you prefer would depend on your a priori beliefs, there being no independent way of proving the real gender identity of anybody. More research on the circumstances might support one or the other theory (maybe you can link to some research that pushes in the other direction, so we can have a scientific look at this). Or you might choose to reject the search for evidence and decide based on prior beliefs, either that all these children really are transgender, or that many of them are likely not to be so. Whatever the underlying facts there could be social or political reasons for preferring one or the other approach.
But, again, where do you find the fraud in all this?
ROGD is a real phenomena. Trans is a delusion which is the hysteria of the time.
Those who promote this trans delusional psychosis are trying to groom more children to become deluded.
Because you are a fraud duplicating the fraud of Litman.